Overview
The transversal team aims at bringing together all competencies from SPINTEC involving spintronic devices nanofabrication, characterization, circuit integration, architecture, and algorithm techniques to implement hardware solutions for artificial intelligence (AI) and unconventional computing.
Spintronic-based multifunctional devices are a substantial opportunity to improve the energy efficiency of next-generation computing hardware. Moreover, this approach allows taking advantage of brain-inspired computing models to deploy cutting-edge neuromorphic algorithms, crossing the gap between current hardware AI implementations and exceptional brain computing ability.
Research topics
Bio-inspired computing
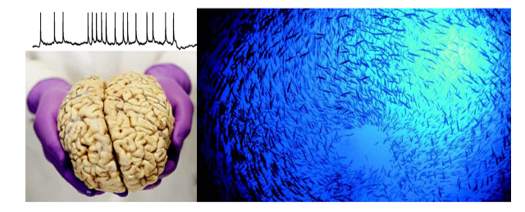
As the brain performs very sophisticated operations and consumes only a few Watts, brain-inspired/neuromorphic computing is a promising path for which spintronic devices can efficiently emulate both neurons and synapses in hardware. Their nanometric size, sensitivity to input stimuli, and interactions make those devices ideal for implementing large arrays of neuro-synaptic elements: spintronic nano-oscillators, spintronic and ferroelectric memristors, magnetic memories, superparamagnetic tunnel junctions, skyrmions, etc.
Probabilistic computing
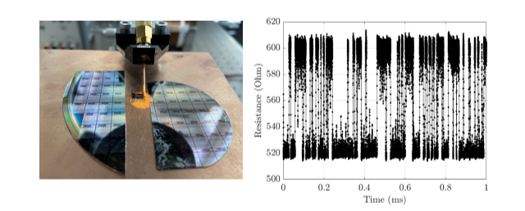
Noise is a crucial ingredient in emulating the stochastic nature of the neural activity and executing energy-efficient computing algorithms such as energy-based or temporal-based machine learning models. In this context, probabilistic computing is a very suitable approach that relaxes usual precision computing constraints. The truly random nature of spintronic devices (such as superparamagnetic tunnel junctions) makes them attractive for hardware implementations of probabilistic computing approaches.
In memory computing
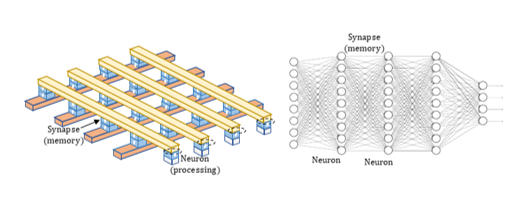
The most promising solutions for non-Von Neumann, in-memory computing architectures are based on the use of emerging technologies, that are able to act as both storage and information processing units thanks to their specific physical properties. High accuracy, Deep neural networks (DNN) can be built with crossbars analog in memory computing concept, involving MRAM families, such as STT, SOT, VCMA, but also with more exotic families of magneto-resistive, and ferroelectric or skyrmion based devices.
The team
Projects
Partners
- CEA LIST
- UMPHY
- CEA LETI
Recent news
- Seminar – Dynamics and oscillations in spintronic neural nets (October 03rd, 2019)
On Thursday October 17 at 11:00 we have the pleasure to welcome Julie Grollier from Unité Mixte de Physique CNRS/Thales. She will give us a seminar at CEA/SPINTEC, Bat 1005, room 434A entitled : Dynamics and ... - Masters thesis projects for Spring 2020 (September 30th, 2019)
You find here the list of proposals for Master-2 internships to take place at Spintec during Spring 2020. In most cases, these internships are intended to be suitable for a longer-term PhD work. Interested Master-1 ... - Seminar – Brownian computing using skyrmions (June 13th, 2019)
On Friday July 12 at 11:00 we have the pleasure to welcome Prof. Yoshishige SUZUKI from Osaka University. He will give us a seminar at CEA/IRIG, Bat 1005, room 445 entitled : Brownian computing using skyrmions We ... - Seminar – Chiral magnetism controlled by spin-orbit torques and spin-torque nano-oscillators: From magnetic memories to neuromorphic computing (May 29th, 2019)
On Monday June 24 at 14:00 we have the pleasure to welcome Jacob Torrejon from SPEC, CEA Saclay, France. He will give us a seminar at CEA/IRIG, Bat 1005, room 445 entitled : Chiral magnetism controlled ... - Masters thesis projects for Spring 2019 (October 10th, 2018)
You find here the list of proposals for Master-2 internships to take place during Spring 2019. In most cases, these internships are intended to be suitable for a longer-term PhD work. Interested Master-1 students are ...